The Transformative Power of Image Datasets for Classification
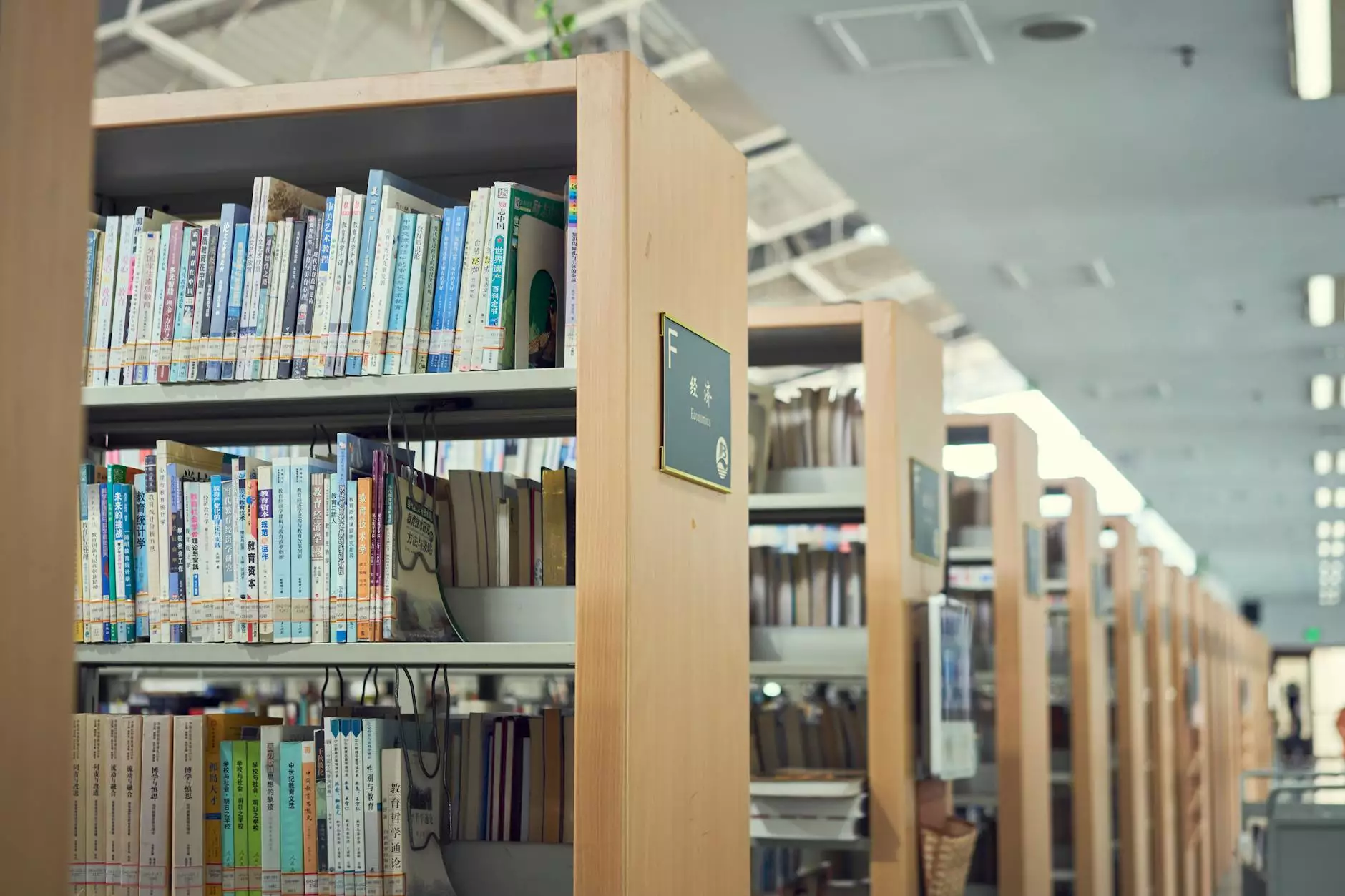
Image datasets for classification play a pivotal role in advancing machine learning and artificial intelligence technologies. As businesses and industries increasingly rely on data-driven decision-making, the demand for high-quality image datasets continues to grow. In this article, we will delve deep into the importance of these datasets, the role of data annotation tools and platforms, and how they can be leveraged to achieve business success.
Understanding Image Datasets for Classification
Image datasets are collections of images that are used to train machine learning models to recognize patterns, objects, and features within images. These datasets serve as the backbone of computer vision applications, enabling machines to interpret visual data similar to humans. Classification is the process where these models learn to categorize images into predefined classes based on their features.
Importance of Quality Image Datasets
The effectiveness of a machine learning model is heavily influenced by the quality of the datasets used for training. The significance of high-quality image datasets for classification can be summarized as follows:
- Precision in Recognition: High-quality datasets ensure that the model can accurately recognize and classify images, leading to better performance in real-world applications.
- Diversity and Coverage: A wide variety of images covering different scenarios, angles, and backgrounds enriches the dataset, increasing the robustness of the model.
- Reduction of Bias: Balanced datasets help in reducing bias, allowing models to perform fairly across various demographics and conditions.
- Faster Training: Well-annotated datasets allow for quicker model training, as the classification process becomes streamlined and efficient.
Data Annotation: The Key to Effective Image Datasets
Data annotation is the process of labeling images within a dataset to provide context and meaning. This is often a crucial step in preparing image datasets for classification. Properly annotated data improves the learning capabilities of machine learning models, allowing them to achieve higher accuracy in classification tasks.
Techniques for Data Annotation
There are several techniques and methods for annotating image datasets, including:
- Bounding Boxes: Drawing boxes around objects in images to identify and categorize them.
- Polygons: For more complex shapes, polygons can be created to accurately delineate object boundaries.
- Semantic Segmentation: Labeling every pixel in an image according to the class of the object it belongs to, allowing for precise classification.
- Image Tagging: Assigning descriptive tags to images based on their content, aiding in the organization and retrieval of images.
The Importance of Using a Data Annotation Tool
To manage the complexities of data annotation, a dedicated data annotation tool is essential. Such tools streamline the annotation process, increase productivity, and reduce human error.
Introducing Keylabs.ai: Your Data Annotation Platform
At Keylabs.ai, we provide state-of-the-art data annotation tools and platforms designed to cater to the unique needs of businesses dealing with image datasets for classification. Our platform ensures that your datasets are meticulously annotated, leading to high-quality outcomes. Here’s why Keylabs.ai can be a game-changer for your organization:
Features of Keylabs.ai
- Scalability: Our platform can handle projects of any size, making it adaptable to your growing needs.
- User-Friendly Interface: The intuitive design of our tools ensures that both technical and non-technical users can easily annotate image datasets.
- Collaboration Tools: We provide features that enable teams to work together efficiently, enhancing productivity.
- Quality Assurance: Our rigorous quality control processes guarantee that the annotated data meets the highest standards necessary for classification tasks.
Benefits of Using Keylabs.ai for Image Classification Projects
Utilizing Keylabs.ai for your image data annotation needs unlocks multiple benefits:
- Enhanced Accuracy: The precision in annotation improves the model's understanding, leading to better classification outcomes.
- Time-Saving: Automating tedious tasks allows your team to focus on higher-level analytic work.
- Cost-Effective Solutions: Our cost-efficient platforms allow businesses to allocate budget more effectively while still receiving quality data.
Real-World Applications of Image Datasets for Classification
Image datasets for classification have a wide range of applications across various industries. Below are some noteworthy examples:
Healthcare
In healthcare, image datasets are crucial for developing and training models that can identify diseases from medical imaging. For instance:
- X-ray Analysis: Machine learning models can be trained to classify X-ray images, helping radiologists in diagnosing conditions.
- Dermatology: Classification models are used to identify skin lesions, improving the speed and accuracy of dermatological assessments.
Automotive Industry
The automotive sector employs image datasets for classification in developing autonomous vehicles:
- Object Detection: Identifying pedestrians, other vehicles, and obstacles on the road.
- Traffic Sign Recognition: Classifying various traffic signs to enhance vehicle navigation systems.
Retail and E-commerce
In retail, businesses utilize image datasets to enhance customer experiences:
- Visual Search: Classifying images allows customers to search for products visually, increasing engagement.
- Inventory Management: Automated classification of product images improves stock management and display organization.
How to Acquire High-Quality Image Datasets for Classification
Acquiring high-quality image datasets can be achieved through various methods, including:
- Publicly Available Datasets: Numerous sources offer free datasets, such as ImageNet, COCO, and Open Images.
- Creating Your Own Dataset: Collecting images related to your specific needs ensures that the data is relevant and useful.
- Purchasing Datasets: Some companies specialize in compiling and selling curated datasets tailored for specific applications.
Considerations for Dataset Acquisition
When sourcing image datasets for classification, keep in mind the following considerations:
- License and Usage Rights: Ensure that the datasets can be used commercially if needed.
- Quality of Images: Assess the resolutions and formats to confirm they meet your project needs.
- Diversity and Size: Aim for datasets that cover a wide range of categories and have sufficient data for training.
The Future of Image Datasets for Classification
As technology rapidly evolves, the future of image datasets for classification looks promising. Here’s what to expect:
- Increased Integration of AI: Advanced AI algorithms will streamline data annotation and enhance classification accuracy.
- Real-Time Data Annotation: Developments in real-time processing will allow for immediate data collection and annotation.
- Ethical Considerations: As discussions around data ethics grow, ensuring fairness and transparency in datasets will become paramount.
Conclusion
In conclusion, image datasets for classification are indispensable in the realm of machine learning and AI. Businesses across various sectors leverage these datasets to enhance their operations and improve their decision-making processes. By utilizing a robust data annotation platform like Keylabs.ai, organizations can ensure the quality of their datasets, leading to successful machine learning implementations. As we continue to innovate and develop, the synergy between data, technology, and business will result in groundbreaking advancements.